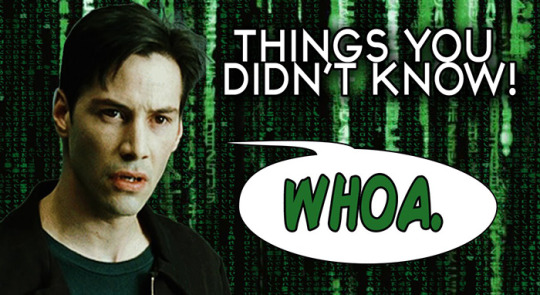
Did you know that there are 1,736,111 Likes on Instagram; 284,722 shared snaps on Snapchat; and 590,278 swipes on Tinder every minute of every day? The growth in the usage of mobile apps and games has created a mountain of data. Millions of users generate significant traces of their behaviour – all of which has value.
Crunching the numbers are the legions of data analysts whose skills are increasingly in demand. The need to find meaningful connections from this data is increasingly putting pressure on these analysts, whose insights and conclusions can affect billion-dollar businesses.
Eating numbers for breakfast
Historically analysts were people with a narrow expertise in statistics and programming. This situation has changed significantly in the past few years as companies have become more aware of the need for people who have one foot in the world of data and the other in the world of business.
The interpretation of the data, the ability to ask the right questions and flexibility in finding answers are the most common problems faced by analysts.
Pavel Trejbal, data analyst at AppAgent – mobile marketing service for gaming studios and startups – has a master’s degree in an experimental field called Cognitive Informatics. His specialization connects technical education with economics, psychology, brain science, linguistics, artificial intelligence and philosophy. “I don’t consider myself as a hardcore expert in any of these fields but the broad knowledge helps me to come up with out-of-the-box solutions of tricky problems.” Pavel explains.
After 6 years in the world of numbers, Pavel has a huge amount of experience, he’s also seen his fair share of poor work and dodgy analysis.
Here are Pavel’s five most common mistakes made by data analysts – and how to avoid them:
1. You believe in perfect algorithms
Focusing on perfecting the algorithm over a simple yet not perfect solution is the most common mistake. You should be fine with less accurate but still very valuable outcomes and insights which your bosses need now, and not in 4 weeks. Getting straight to the point with some error margin is usually more effective. Time matters in business!2. You believe in best practices
Don’t! Every business and task is different. Best practices can be a great inspiration but you should always think about your own solution. Don’t rely on similar cases, be open to study and analyze things as you would do if approaching the task for the very first time.3. You blindly believe your data
If you find some interesting significant patterns, use the rule of 4 eyes. Consult your hypothesis with the product management, community managers or game designers. These are people who, quite simply, understand users and the product better than you. Often it’s a wrong interpretation of the behavior or there’s a technical error in the data.4. You don’t clean data properly
Data cleansing is usually the boring part of data analytics and it often takes up the most time – but it is worth of it. You will understand what is missing or wrong and what are the existing limitations for interpreting your analysis. If you skip this step the analysis will be polluted and insights could be absolutely wrong. Remember a simple rule: garbage in = garbage out.5. You don’t understand differences in tools and metrics
Each analytical tool is specific due to different technological solution or different metrics definition. Make sure that you are aware of them! Recently we evaluated A/B test using KPIs like conversions and revenue with Google Analytics Sampling Method. First the variant A performed much better than the variant B in both metrics. We didn’t trust it, downloaded the raw data and performed the analysis manually to avoid sampling. The conclusion was the absolutely opposite, the variant A was worse in both metrics.
Get up from your chair
Pavel is 100% certain that, no matter if the analyst is internal or external, he or she shouldn’t sit in an “ivory tower” away from the daily business of the company. On the contrary, the role should be involved in the key management, marketing and product meetings.
That’s how analysts could understand needs of decision makers, soak up product information and could propose how data can help the company. A secondary positive effect is that decision makers will better understand the value of analytics in games and apps and will evangelize new outcomes and learnings through the company.
Analysis is an essential part of the process and shouldn’t be ignored. It’s a complicated process, but followed logically shouldn’t pose too many difficulties. And remember, we’ve made the mistakes so you don’t have to.